Digitally Enhanced Advanced Rail Signalling Services (DEARSS)
The project will conduct a case-based and action research, with the aims to understand the existing business model, delivery system and opportunity space for the London Underground and National Rail services, to design proof-of-concept DEAS business models; and to use Data Envelopment Analysis to analyse the proposed business model and delivery system and provide a proof-of-concept.
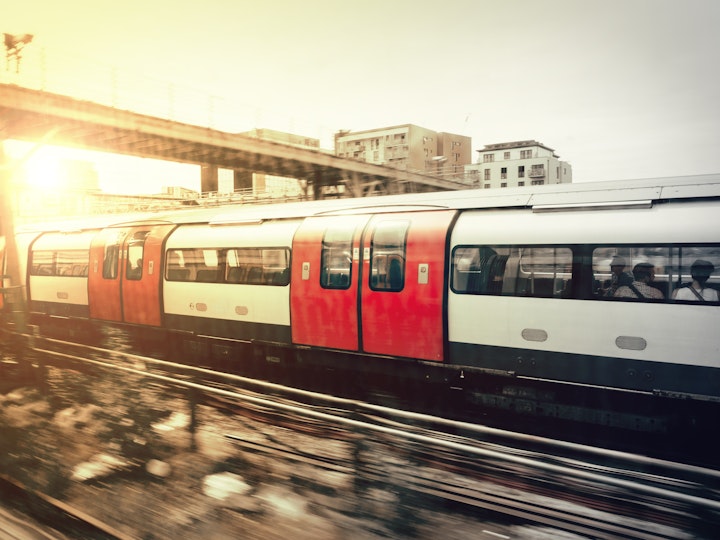
Urban population growth means that 66% of the population is expected to live in cities in by 2050. The 2019 Williams Rail Review[1] proposes that rail will be key for the efficient movement of people living in urban areas. With the future of UK transport heavily reliant on rail it is crucial these transport systems are reliable.
Recent advances in technology and investments in infrastructure has enabled the UK rail industry to increase volume, improve visibility and adjust to variation of demand. Whilst improvements have been made, delays and lost customer hours are still increasing. Between April 2019 and November 2019 26,573,334 customer hours were lost on the underground compared with 19,371,514 in the same period for 2018[2]. Given demand is set to increase further, and volume increases directly affecting train punctuality (Coxon et al, 2015), work is needed to improve the system performance of the rail networks, above and below ground.
Digitally Enhanced Advanced Services (DEAS) are expected to help improve performance, maximise productive capacity and open additional revenue streams. Few examples of DEAS exist in the rail industry, e.g. Alstom’s availability contract with Virgin’s West Coast mainline trains. Investigations are needed into how digital technologies can enhance business model design, impacting how business capabilities and engineering (including human factors) functions can be combined to improve performance and experience with rail transport systems.
Given the potential benefits of DEASs, our project Partner, an electronic systems and service provider, is currently investigating how they could deliver a performance-based DEAS for their signalling and traffic management systems for the London Underground (below ground) and National Rail services (above ground). To do this, they have partnered with our multidisciplinary team to understand and develop a performance-based DEAS and provide a proof-of-concept using data currently available within their operations. To begin, performance is defined as providing a specified level of reliability for their signalling and traffic management systems. This definition will evolve during data collection. Based on these requirements, the proposed research conducts a novel case-based and action research project. The research aims are:
- Understand the existing business model of our project partner, delivery system and the DEAS opportunity space for the London Underground and National Rail services.
- Design proof-of-concept DEAS business models for our project partners signalling and traffic management systems for the two cases outlined.
- Use Data Envelopment Analysis to analyse the proposed business model and delivery system and provide a proof-of-concept of the performance-based DEAS for our project partner.
The project is in collaboration with the University of Exeter, University of Surrey and Coventry University, and has a total Value of £97,949.
Funded by: EPSRC Digitally Enhanced Advanced Services (DEAS) Network+
Principle Investigator: Dr Phil Davies (Henley Business School)
[1] Williams Rail Review: https://assets.publishing.service.gov.uk/government/uploads/system/uploads/attachment_data/file/802472/rail-in-the-future-transport-system.pdf
[2] Lost customer hours are lost due to disruptions of <2 minutes on the London Underground. Data available here: https://data.london.gov.uk/dataset/london-underground-performance-reports
Authors |
---|
This site uses cookies to improve your user experience. By using this site you agree to these cookies being set. You can read more about what cookies we use here. If you do not wish to accept cookies from this site please either disable cookies or refrain from using the site.